by Tobias Kuhn, Vrije Universiteit Amsterdam, NL
With the demonstration earlier this year that scientific articles can be formalized and therefore machine-interpretable, I provided background to this process in a blog post that reports on work with Cristina Bucur, Davide Ceolin, and Jacco van Ossenbruggen [1,2], and it is shared here as a follow-up to the presentation I gave at the IOS Press symposium.
This post originally appeared on the Github website here.
I believe we have made the first steps venturing into a new era of scientific publishing. Let me explain. At what point exactly a new era begins and what counts as first steps are of course subject to debate. There is therefore a section listed at the end of this piece covering related initiatives and further reading.
Science is nowadays communicated in a digital manner through the internet. We essentially have a kind of "scientific knowledge cloud," where researchers with the help of publishers upload their latest findings in the form of scientific articles, and where everybody who is interested can access and retrieve these findings. (This is in fact only true for articles that are published as open access, but that is not the point here.)
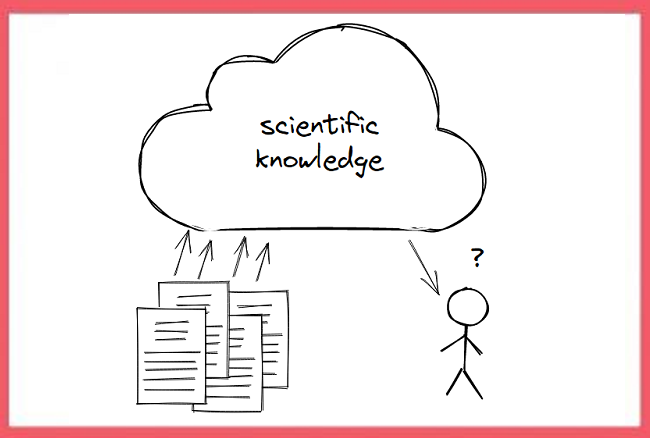
In a sense, this scientific knowledge cloud has been a big success, but it also has serious limitations. Imagine somebody wanting to build an application that feeds on all these findings, for example to help researchers learn about interesting new developments or to summarize scientific consensus for laypeople. Concretely, a developer might face a task like this one:
Retrieve all genes that have been found to play a role in a part of the respiratory system in COVID-19 patients. Only include results from randomized controlled trials published in the last 7 days.
To a naive developer without experience in how scientific knowledge is communicated, this might sound quite easy. One would just have to find the right API, translate the task description into a query, and possibly do some post-processing and filtering. But everybody who knows a bit how science is communicated immediately realizes that this will take a much bigger effort.
Why text mining is not the solution
The problem is that scientific results are represented and published in plain text, and not in a structured format that software could understand. So, in order to access the scientific findings and their contexts, one has to apply text mining first. Unfortunately, despite all the impressive progress with deep learning and related techniques in the past few years, text mining is not good enough, and probably will never be.
To illustrate the point, we can look at the results of the seventh BioCreative workshop held in November 2021, where world-leading research teams competed in extracting entities and relations from scientific texts. Just to detect the type of a relation between a given drug and a given gene out of 13 given relation types, the best system achieved an F-score of 0.7973 (Figure 1), from this BioCreative workshop paper (PDF).
Figure 1: Ascertaining the F-score relation type